The project SurfAIce aims to exceed current metal cutting standards
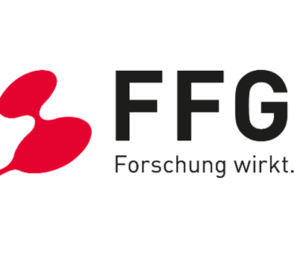
The project SurfAIce is funded by the Austrian Research Promotion Agency (FFG) under the project number 41862958.
Details
The project SurfAIce aims to exceed current metal cutting standards by developing an Artificial Intelligence (AI) application for surface-roughness prediction. The model resulting from this application will allow for automated planning of manufacturing strategies and cutting parameters. Exceeding current standards is achieved by relating research on chatter to the work on surface roughness as well as connecting sensor information to meta information from CAM and CNC control systems. This data will be utilized in machine learning models for surface roughness prediction and enable the machine tool, not only to predict target parameters but also dynamic and complex machining behavior. The result of the project will be a first prototype of a self-learning machine tool that is able to identify optimal manufacturing strategies and cutting parameters with an output of the expected surface roughness of the part.
Flexible production of small lot sizes is equally demanding for manufacturers and demanded by customers. It is demanding because manufacturers struggle to provide automated and efficient planning and execution systems for a lot size of one. It is demanded by customers due to the flexibility of production on one hand, and the climate crisis and the ensuing need for ecological and economic production on the other hand.
To map the complexity of small lot sizes, the project “SurfAIce” was brought to life. The aim of SurfAIce is the development of promising Artificial Intelligence (AI) applications by developing a model for AI-based surface-roughness prediction for automated planning of manufacturing strategies and cutting parameters. This is one of the first and most crucial steps toward a self-learning machine tool, specifically for surface roughness prediction and optimization.
In reaching this goal, it is important to relate the previous analyses on chatter phenomena, the respective cutting parameters, and machine dynamics to the surface roughness. It also requires the connection of sensor information to production meta information from CAM and CNC control systems. Furthermore, machine learning methods have to be utilized for the prediction of surface roughness. This should not only result in the prediction of target parameters that have been developed previously but the prediction of dynamic and complex machine behavior. In this way, machine learning is incorporated into a CAD/CAM engineering chain.
The result of the project will be the first prototype of a self-learning machine tool that is able to identify optimal manufacturing strategies and cutting parameters with regard to the expected surface roughness of the considered part. Such a machine tool demonstrates how an initially provided manufacturing plan can be updated and automatically fed back to the CAM system.
ModuleWorks is mainly engaged in the CAM planning prototype development. This involves the identification of machining rules for surface roughness control strategies, the implementation of machining rules in a software module and interlinking with CAM planning software as well as a data visualization tool for human operator assistance, and the development of a prototype system for laboratory function tests.